TopoAI: Topological Approaches for Improved Structural Correctness
- Alexander Spiridonov ETH Zurich
- Alexander Veicht ETH Zurich
- András Strausz ETH Zurich
- Richard Danis ETH Zurich
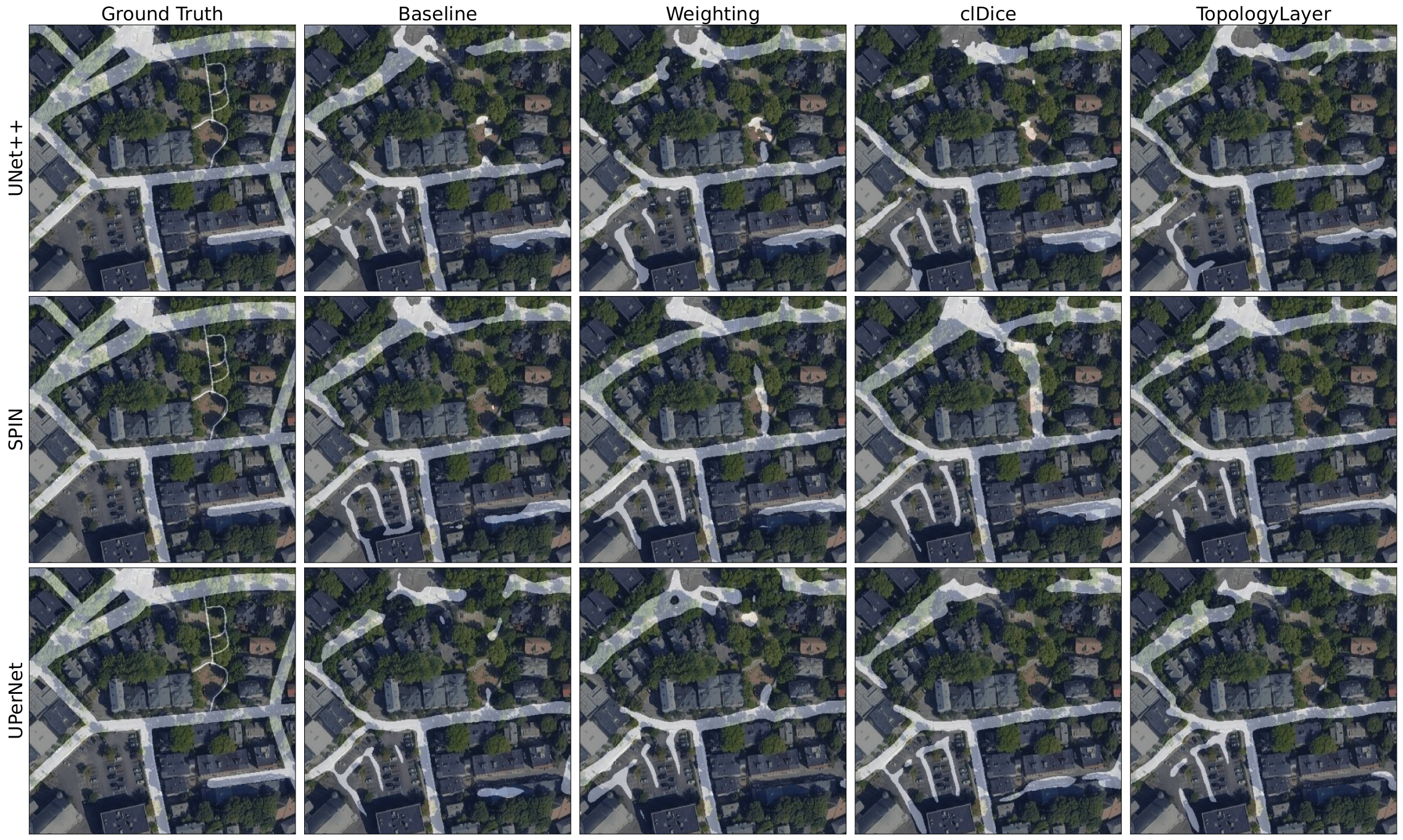
Overview of the different segmentation masks for all our models and losses.
Abstract
Road network extraction from satellite images is a critical task for various downstream applications such as route planning and autonomous driving. While state-of-the-art methods based on deep learning have shown remarkable performance in terms of accuracy or IoU, even minor prediction errors can lead to disconnected roads, impacting certain use cases such as route planning. In this study, we investigate this issue across multiple models, revealing the presence of incorrectly disconnected sections in predicted masks. To address this challenge, we propose three different approaches based on regularization and re-weighting. First, we introduce a novel weighting technique that leverages the inherent properties of the roads. Drawing inspiration from the medical domain, we explore both implicit and explicit measures for topological correctness, namely Soft-clDice and TopologyLayer. Through extensive evaluation, we demonstrate modest yet significant improvements in topological correctness. Our best results are achieved using soft-clDice, yielding an enhancement in topological correctness, measured by clDice from 0.814 to 0.844.